
Conference proceeding
G. Weiss and M. Bajec, “Discourse Sense Classification from Scratch using Focused RNNs,” in Proceedings of the Twentieth Conference on Computational Natural Language Learning - Shared Task, 2016, pp. 50–54.
Abstract
The subtask of CoNLL 2016 Shared Task focuses on sense classification of multilingual shallow discourse relations. Existing systems rely heavily on external resources, hand-engineered features, patterns, and complex pipelines fine-tuned for the English language. In this paper we describe a different approach and system inspired by end-to-end training of deep neural networks. Its input consists of only sequences of tokens, which are processed by our novel focused RNNs layer, and followed by a dense neural network for classification. Neural networks implicitly learn latent features useful for discourse relation sense classification, make the approach almost language-agnostic and independent of prior linguistic knowledge. In the closed-track sense classification our system achieved overall 0.5246 F1-measure on English blind dataset and achieved the new state-of-the-art of 0.7292 F1-measure on Chinese blind dataset.
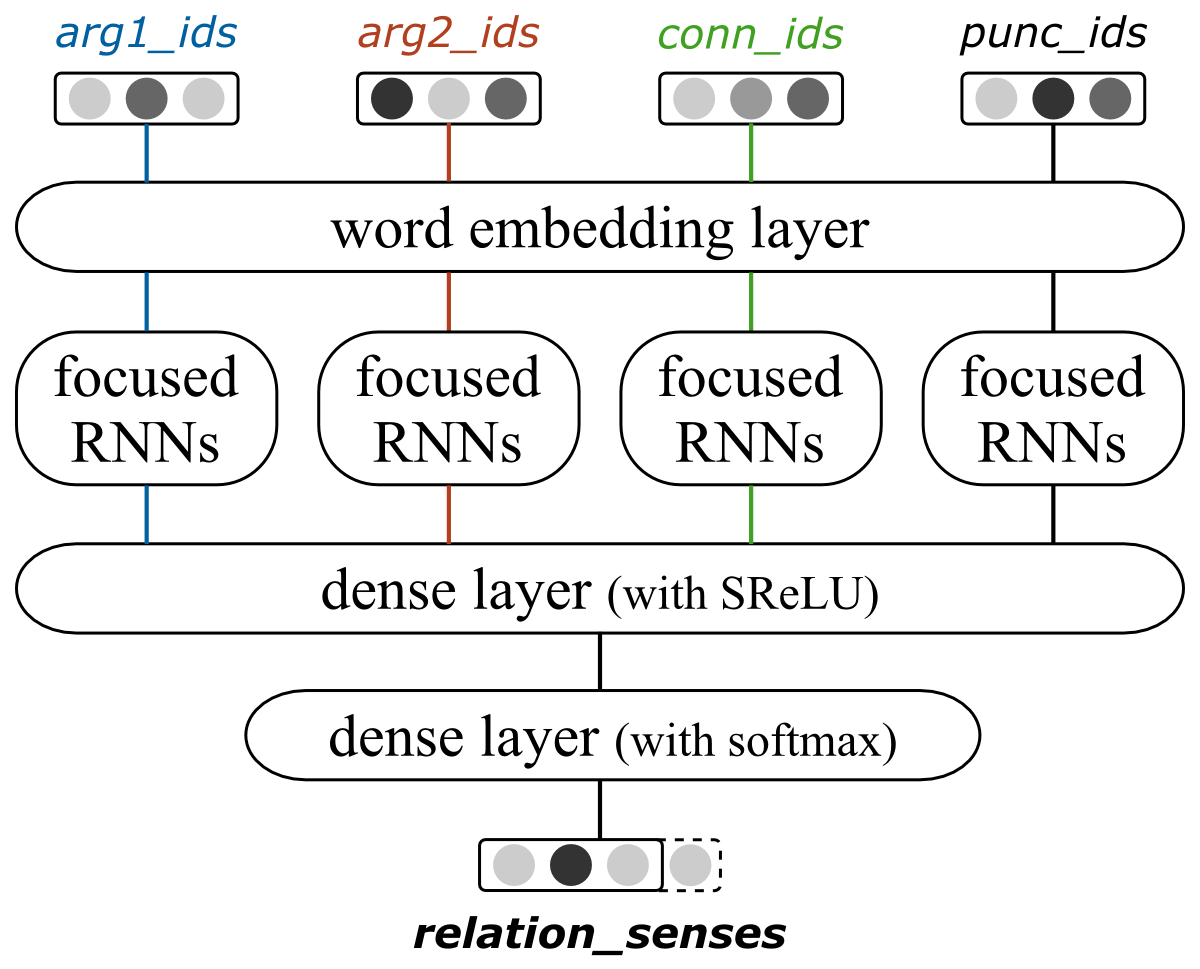