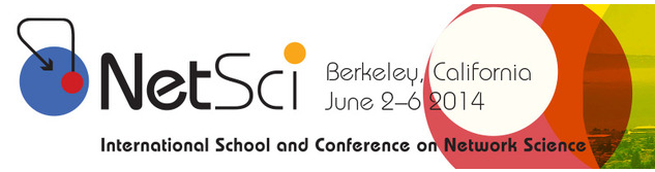
Conference proceeding
L. Šubelj, G. Weiss, N. Blagus, and M. Bajec, “What coins the bitcoin? Exploratory analysis of bitcoin market value by network group discovery,” in Proc. of NetSci’14, 2014, vol. 105, no. 2008.
Abstract
Bitcoin is a peer-to-peer digital currencies that gained tremendous popularity in recent years. Daily market trade currently exceeds 100000 BTC (over 50 million USD) with 70000 processed transactions. It relies on a network of computers that solve complex mathematical problems as a part of a process that verifies and permanently records every transaction made. Unlike traditional currencies, no central authority governs the supply or has control over bitcoins. The bitcoin market value thus depends solely on people’s confidence or trust in it, similar to other real-world commodities and assets. Consequently, little is known about the trading behavior on exchange markets and their periodic or mutual dynamics. We thus here report the results of a preliminary exploratory analysis of bitcoin market value from a popular exchange market BitStamp. We collect the data for a period of five days in January 2014 at a rate of about one minute and construct different network representation of the time series. In particular, we consider proximity networks including cycle and correlation networks, transition networks and visibility graphs. Cycle network has a grid-like structure with uniform degree distribution and high clustering. On the contrary, the transition network reveals very sparse topology with bell-shaped degree distribution and no pronounced degree correlations. Correlation network and visibility graph are scale-free and small-world with assortative mixing by degree, otherwise a characteristic of social networks. Next, we apply a node group discovery approach to constructed networks in order to gain an insight into the their structure, and to reveal possible periodic patterns and other dynamics of the time series. In fact, complex networks methods provide knowledge that is complementary to that of the standard approaches of time series analysis. We adopt group detection framework that can detect densely linked groups of nodes known as communities, groups of structurally equivalent nodes denoted modules, and different mixtures of these, with core/periphery and hub & spokes structures as special cases. According to the above, cycle network is too dense to reveal any clear structure. On the other hand, most significant groups in transition network are communities with high modularity that represent the quantiles occupied by the bitcoin market value for some period in time (6 hours). The latter could be used for concept drift detection, which is a difficult problem in stream mining. Groups in correlation network are core/periphery-like structures with negative modularity, where dense core correspond to periods in time with consistent bitcoin behavior and periphery are notable shifts in the value that appear rather periodically. Whether this could be adopted for market price prediction remains unclear. Finally, groups in the visibility graph show similar characteristics with periphery corresponding to local extremes in the value that dominate a certain period of time. The above network representations can also model multidimensional times series, which enables the analysis of bitcoin market value and trade from several exchange markets simultaneously. Since the value can differ substantively across the markets, predicting the future fluctuations at one market from the dynamics of another could be of considerable practical value.