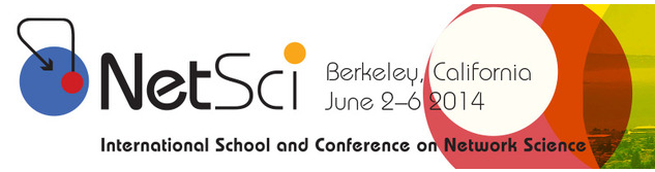
Conference proceeding
N. Blagus, L. Šubelj, G. Weiss, and M. Bajec, “Large networks grow smaller: How to choose the right simplification method?,” in Proc. of NetSci’14, 2014.
Abstract
Network simplification proved as an effective tool for reducing large real-world networks and at the same time providing for sufficient fit of original network. However, even though a number of analyses have been performed observing the changes of networks under the simplification, broad understanding of the whole process remains only partial. The questions such as ‘How to compare original (i.e., complete) and simplified (i.e., incomplete) network?’, ‘What factors impact the effectiveness of simplification process?’, ‘What size of simplified network provides for the best fit of original network?’, ‘What simplification method to use?’ are far from solved in the literature. In our study, we analyze over 30 real-world networks of different size and origin (e.g., social, information, technological). We reduce networks with several simplification methods (e.g., random node and link selection, breadth-first sampling, merging based on balance-propagation) and observe the changes of several fundamental properties (e.g., degree distribution, clustering coefficient, degree mixing, and density) under simplification. We show that the reduction on about 10% of original network provides for adequate preservation of important properties. The best performing methods prove to be random node selection based on degree and breadth-first sampling. The results also show the size of simplified network influence the effectiveness of simplification method, while the size and type of original network do not. Besides basic properties, we explore also the changes of network structure under simplification. Particularly, we focus on different groups of nodes, commonly observed in real-world networks (e.g., communities, modules and mixtures of the two). In this case, the changes in the simplification effectiveness occurs among different types of networks. For example, simplified social networks exhibit even stronger community structure than original networks, while in simplified information networks the number of mixtures increases. However, in general, the proportion of nodes explained by the group structure enlarge in sampled networks, moreover the goodness of the preservation of node group structure does not depend on the choice of the simplification method. To summarize, the main advantage of our analysis is large number of networks considered. Therefore we provide for reliable results concerning the effectiveness of the simplification process and support a better understanding of the changes of networks under the simplification process. In our future work we intend to create a framework for adaptive simplification of real-world networks, which would suggest the best simplification method for a given network based on its properties and the further use of the simplified network.